Use of Unmanned Aerial Vehicle (UAV) Imagery to Monitor Progress of Early Forest Ecosystem Restoration in an Opencast Mine
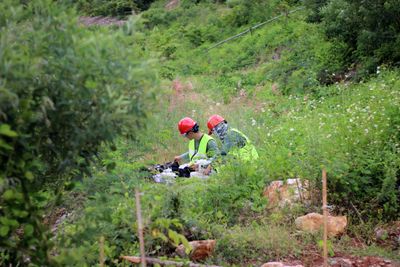
Changsalak, P., 2022. Use of unmanned aerial vehicle (UAV) imagery to monitor progress of early forest ecosystem restoration in an opencast mine. MSc Thesis, The Graduate School, Chiang Mai University.
ABSTRACT: Monitoring forest restoration is essential for improving and advancing restoration techniques, but human-based monitoring is costly as it requires intensive labour in the field. Although aerial images from unmanned aerial vehicles (UAVs) could potentially replace labour, aerial monitoring of newly planted trees is challenging because of small tree sizes, especially during the planting and initial growing stage. This research developed and tested an aerial technique to monitor survival and growth of young trees, which were planted to restore an open-cast mine. A quadcopter with 20-megapixel RBG camera was used to capture tree growth in the planted sites from 10 m above ground, every 3 months over the first year after planting. Tree variables, derived from photogrammetry (orthomosaic images and 3D point cloud software) were compared with conventional ground-survey measurements. Three photogrammetric software tools for this purpose were compared, DroneDeploy outperformed trial versions of 2 other software in terms of producing 3D point clouds and preliminary manual sapling height measurements. It therefore was used to perform the study. Orthomosaic images and 3D point clouds were able to detect rates of survival saplings up to 85% and 64 %, respectively. Tree-height measurements from imagery correlated well with ground-survey measurements (R2 = 0.57, P< 0.001) with a moderate correlation, while crown area measurements (both methods) correlated with image-based measurement (R2 = 0.62 and 0.68, P< 0.001), after the trees had been growing 1 year. Correlation of tree root-collar diameter predictions from image-based height was low (R2 = 0.36, P< 0.001), after the trees had been growing 9 months. Reliability of tree detection and measurements increased during the second rainy season after planting, when most of the trees had grown taller than 0.8 meters. At present, monitoring progress of newly planted trees is more accurate by ground than by drone surveys. However, the study showed increased correlation of drone surveys after 1 year’s growth. The study shows that seasonality, species traits, appropriate age and size of the target saplings all need to be considered during development of appropriate aerial-based techniques, to monitor the progress of forest ecosystem restoration sites of greater complexity. Differences between drone-acquired and ground data, when ranking species by relative performance index were large. Improvements in the collection and analysis of image-derived data will be needed, before aerial base species-selection decision making becomes reliable, particularly application of AI technologies to replace manual measurements. However, once such developments are achieved, it is likely that image-based methods to monitor saplings in the early stages of forest restoration will become a viable alternative to ground surveys